The Invisible Interface
Written by: Mark Nguyen
Revised by: Brice Gower, Anon 1
Last revised: Mar 15, 2025
Labels: Human Written, Exploratory Brief
Topics: AI, Science Fiction, UX, AI, Consumer Behaviour
7 Key Ideas:
- Americans are generally pessimistic about AI.
- Science Fiction is great for explaining, and examining Western user attitudes, conducting strategic foresight exercises, and producing inventive UX, UI, and Interaction Designs.
- Scientists have conceptualized of the “invisible interface”, or “Zero UX” for decades.
- Users want easy to use interfaces, but AI interfaces also have to be transparent.
- Trust related UX improvements within the customer service (CX) agent category can save $X00’s of millions, with plenty of relatively simple fixes.
- Major UX related discoveries for Multimodal Generative AI interfaces will likely come from, or be heavily inspired by the Ecological Sciences.
- Major UX inventions for Multimodal Generative AI interfaces will be experienced within 3 to 7 years.
Preamble

Americans are mostly pessimistic about AI. The Pew Research Center’s 2024 study on attitudes on AI reveals that American attitudes are predominantly pessimistic, Whereas Chinese attitudes are primarily optimistic [1]. This may appear strange, as the technologically oriented, R&D focused economies are quite similar. Both share a focus on tech R&D, STEM education, lead investments into tech, and maintain accelerationist consumption cultures. So, why the contrast in attitudes about AI?
Hint: It’s in our media. In our 2024 research, we’ve offered a partial explanation to this contrast, being attributable to decades of dystopian characterizations of machine intelligence in contemporary Western science fiction.
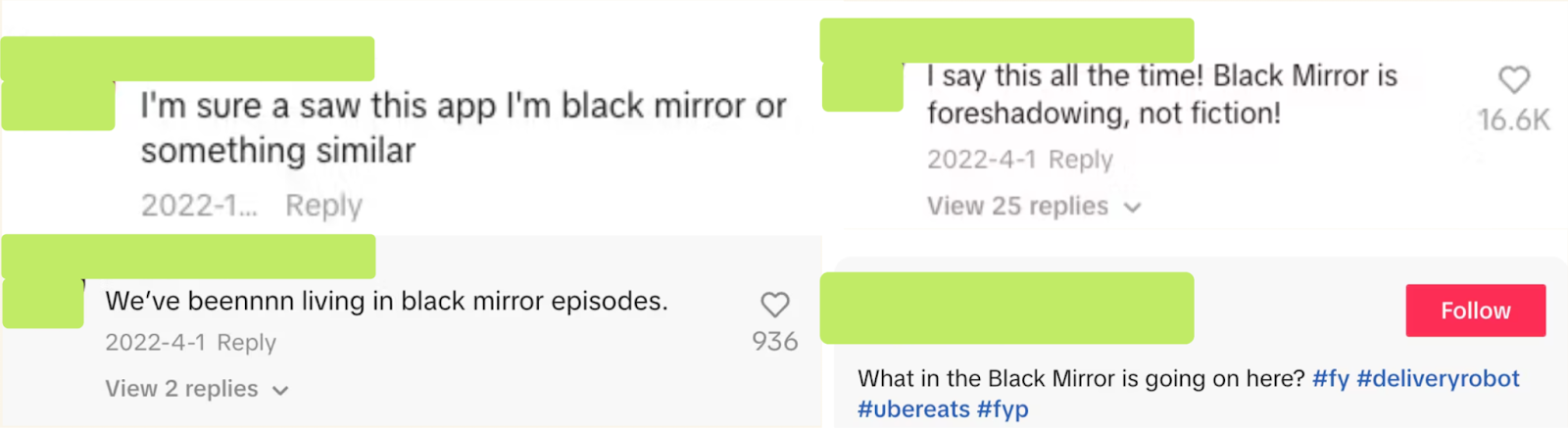
“This is straight from the show Black Mirror” - Reddit Comment, in reference to a recent release from Open AI
Although the science-fiction genre is typically unassociated with “serious” technology product development, it maintains a strong yet quiet influence on consumer attitudes. We found that it defines much of the market’s consumer dynamics. While it is not the largest, nor it is the fastest growing media genre [2, Statista], speculative science fiction works such as the Spike Jonze directed movie Her, Alex Gardland directed Ex-Machina, and classics like the Terminator 2, disproportionately shape attitudes, and perceptions. There is longstanding research in consumer behaviour that such attitudes and perceptions ultimately influences behaviours in new technology adoption [2].
In 2023, when we had an opportunity to work with a now Microsoft Acquired Consumer AI firm, we studied the impact of over 10,000 science fiction assets on consumer attitudes, distilling 7 key representations of the human-robot relationship. From this extraction, we were able to synthesize over 50 novel product improvements, spanning transparency, trust, and safety. Two of our three key recommendations are now widely expressed by the consumer LLM market [3]. This working whitepaper includes some of this research’s output. Science Fiction continues to create a rich site of study, novel source for IP development, and a useful lens for understanding consumer attitudes.
What did we find?
A theme that we encountered was what was imagined earlier by Human Computer Interaction scientists as the “invisible interface”. Put simply, the idea describes a collection of interaction experiences with robots, machines, and AI that is so seamless and natural, that the technical interface disappears from user perception.
Dreams of the Invisible Interface
[Design, Science Fiction]

Over the decades, many have written about this ideal of "Ubiquitous Computing”. Technologist Mark Weiser (1953-1999) first coined this term in 1988 while leading Xerox Parc, the birthplace of the Graphical User Interface (GUI) - and somewhat of a Garden of Eden of UX [3]. He, among other anthropologists and media theorists, describe language and writing itself as the first information technology, and how its disappearance from awareness, allowed us to “focus beyond them on new goals” [4, Scientific American].
Influential writing by HCI Pioneer Don Norman in The Design of Everyday Things, as well as the prominent example of the late Steve Jobs, further pursued this invisible interface. Steve’s bold design direction for Apple, and hardware design decisions focused on ease of use, made this ideal, a reality. British science fiction writer Arthur C. Clarke’s articulation that “any advanced technology is indistinguishable" from "magic” frequently circulates tech Twitter in the startup community, further idealizing the intuitive, and seamless user experience amongst technologists. The important 2003 Technology Acceptance Model (TAM) paper by Paul Legris claimed that a technology’s perceived ease of use can explain up to 40% of the adoption of a system [5, I&M].
With the recent rapid growth of accessible consumer-grade LLMs, the ideal of an invisible interface is becoming increasingly achievable. Consumer LLMs exemplify this shift: grounded in the familiar conversational interactions most internet users already know well, the interface effectively fades into the background. Yet this transition is not without challenges. These AI-driven interactions have created new, unique UX expectations—that current interfaces do not yet reliably fulfill. Moreover, measuring user experiences in conversational AI remains difficult.
How do we measure this new “invisible UX”, when it comes to AI interfaces?
The non-deterministic nature of consumer AI experiences can complicate. Provided the same, default state, ChatGPT may produce different outputs provided the same user text input. By default, LLMs use a random sampling strategy, rather than deterministically selecting the most probable token. This means that LLMs can give you a different answer, each time. This engineering decision, whilst improving the user experience, may make accurate measurement, a core concern of human factors research, more difficult.
Traditional usability measures adapted from military technology human factors studies such as the SUS-PR, were repurposed for the GUI based web interface era. Now, this has been updated to the BUS-15 (Bot Usability Scale, 15 Questions), for conversational AI interfaces [6]. Despite this, it's not clear whether these capture spectra of potential consumer expectations and experiences. In the case of customer service bots, simple Issue Resolution Rates (e.g. “Did I resolve your issue today? Y/N”) may be a more direct and useful method of measuring the user experience than traditional navigation based usability measures. For example, what if a tired new mother uses a Lululemon Chatbot, to vent about her seemingly unrelated caregiving duties? How is this long tail of potential user experiences to be measured and evaluated, as both user expectations and multi-agent, multimodal, and the multi-everything exponentiate?
Late in 2024, Jakob Nielsen, of N/N Group, proposes an “intent based interaction” paradigm [7, Jakob Nielsen], which appears to be the best way to evaluate these interfaces. Nielsen this "invisibility" of interfaces. This approach considers the long tail of possible inputs, and randomly sampled outputs of most default state consumer LLMs. Despite the effectiveness of the simple text based conversational interface in disseminating generative AI (cheap & fast) the design community has continually called for innovations in the generative AI user experience. The clearest potential contributors are found in multimodal interaction, where voice, text, and images are both inputted and outputted by human users. Research on how multimodal generative AI interactions impact user behavior is new, but does exist. [8, Personal & Ubiquitous Computing] Overall, Nielsen’s proposed measures, and the innovations in multimodal interfactions to which this whitepaper is not primarily focused on, demonstrate changes towards new interactions, and interaction evaluation.
Users want invisible, but also transparent interfaces.
[Cognitive Science, Interaction Design]
In addition to easy to use interfaces, users now expect transparency. A skyrocketing level of industry investment into Transparent AI, the volume of academic literature on consumer Trust in AI, and as well as several key consumer trust surveys, demonstrate a growing demand for transparency in AI products. In 2023, we developed a set of recommendations that addressed these transparency gaps. We were able to identify several UI/UX elements that could increase perceived transparency in GenAI, which are now commonplace.
This class of improvements that follow these recommendations, we believe, are of the largest possible UX improvements available in the customer service AI agent category. This is significant as customer service agents are the largest contributor of revenues (~38%+) within the Gen AI implementation services market [9, Mckinsey & Co]. With this considered, our estimate is these UX improvements we propose can track to the low hundreds of millions of $US dollars in value globally, within the next 3 years.
These improvements in transparency were in part based on UX heuristics developed by human computer interaction scientist and co-founder of N/N Group, Jakob Nielsen (Now UX Tigers). One we will explore is “visibility of system status”. Visibility of system status, as invented and described by Nielsen Norman Group, is fundamentally about communicating information about the system so that users can make better decisions [10, N/N Group].
Visibility of System Status Example: Loading Bars
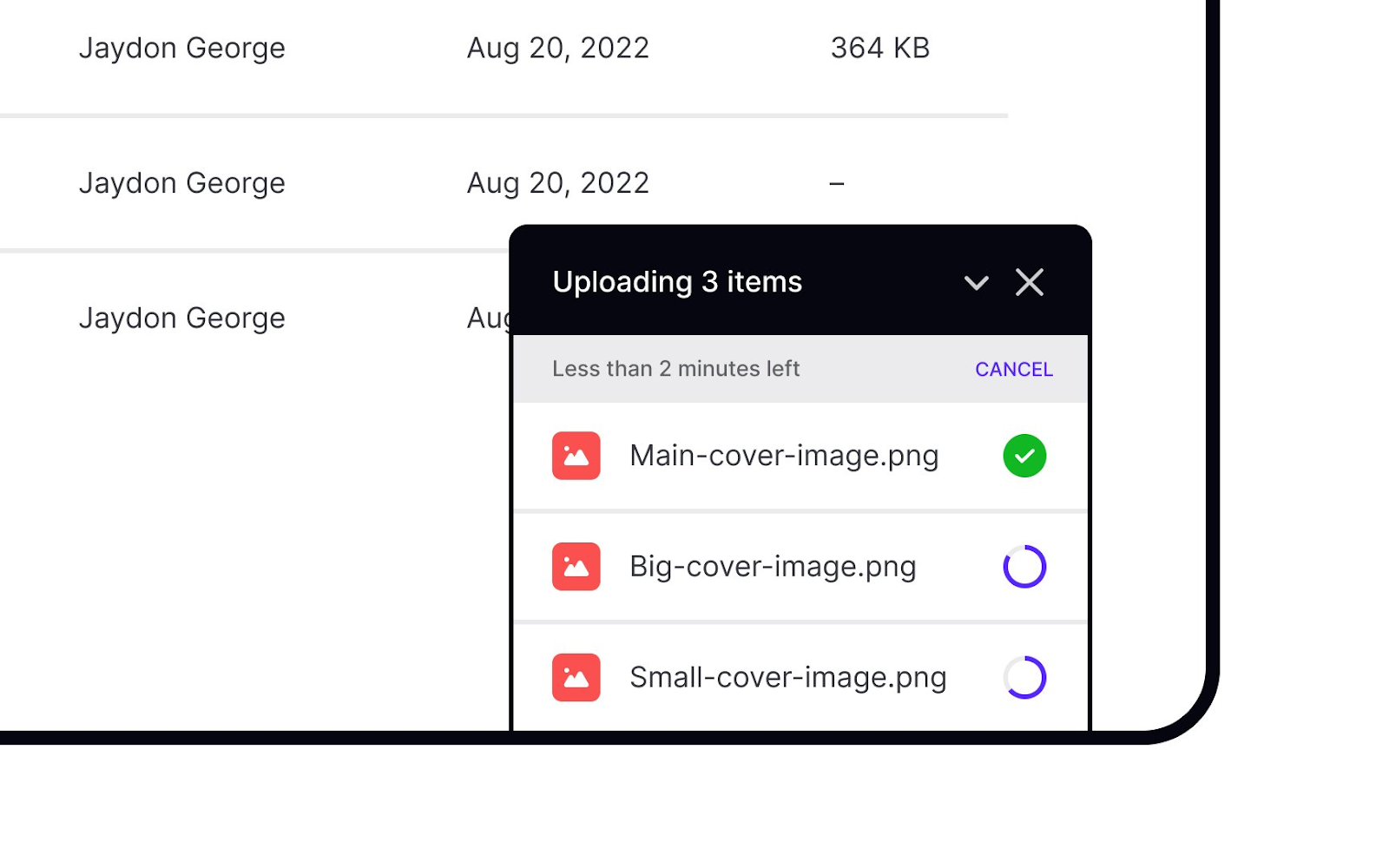
When it comes to customer service AI chatbot experiences, the industry hasn’t yet been able to effectively communicate information on the system. RAG based customer service agents don’t communicate their capabilities up front. They even more rarely communicate the data sources that are accessed when returning a result. They almost never communicate their internal training processes. How would a user know whether or not the bot could be trusted?
Think of it like this: Just as you might expect signals of quality visiting some high end establishment, one should expect a chatbot to quickly signal its quality, or more specifically, what it can do for you. Its “affordances”. Many current AI based customer service systems don’t clearly communicate what they can do for you. Major advantages in this category may come from companies that were able to effectively communicate agent’s abilities. We are already beginning to see this.
Case example: DeepSeek. DeepSeek gained attention for being the first to release “chain of thought” reasoning, which developers claim display the model’s internal thinking process.
Increasing interface Transparency: CoT reasoning
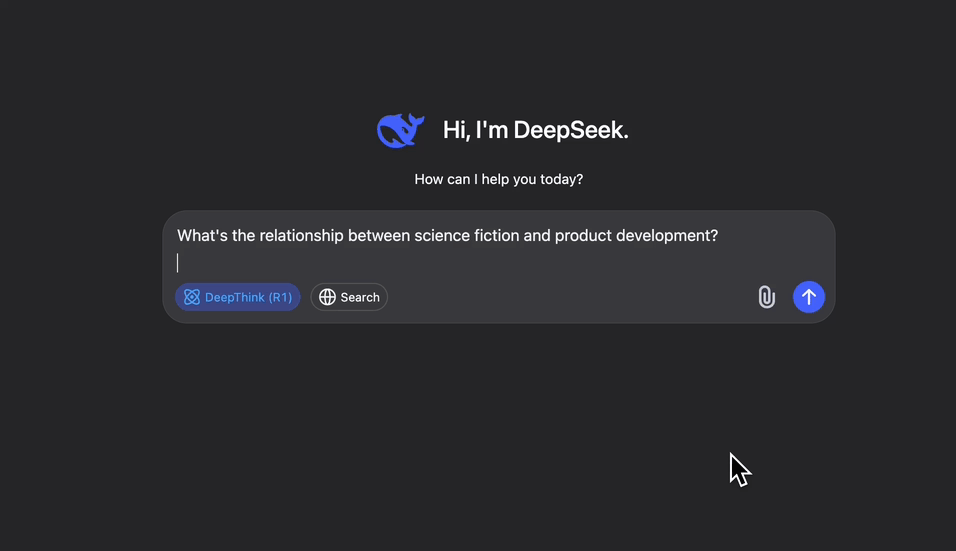
Transparency increasing AI does not need to be perfectly accurate, to be useful.
While this new interaction isn’t accurate in representing the internal processes [11, NeurIPS], DeepSeek’s widespread adoption, and the fast follow-on CoT releases by competitor firms demonstrates to us that explainable AI elements don’t necessarily need to be perfectly accurate to be useful. Research on explainable AI furthers our stance, as the range of xAI solutions that are more technically accurate, but less human-friendly, have found little adoption [12][13].
An irresistible rabbit holing into Donald Hoffman’s interface theory of perception presents a similar argument. Hoffman hypothesizes that humans have evolved to perceive less truth, instead evolving in directions that provide greater control over its environment. So the argument for a truthful blackbox, as poised by OpenAI researchers (Shavit et al) in OpenAI’s 2024 open research paper, technical accuracy of the model’s operation may not necessarily be required to improve the user experience [14, OpenAI]. Still, researchers at IBM, among many other open source developers have formed an opinion around as a factor that produces trust [15, IBM Research].
The most truthful view into these models is indeed provided, but just not always served by the end user interfaces themselves. This transparency is served through other means: research papers, media reporting, and even YouTube explainer videos by Ben1Brown. We have produced UX flows that increase transparency consequently, trust, into user facing AI deployments, particularly for customer service and RAG based applications.
Reliability still matters.
[Consumer Behaviour, Interaction Design]
In order for these interfaces to be truly invisible, it also requires a trustworthy technology that is sufficiently reliable.
Reliability = How often does this perform as expected?
Increase reliability, by making sure user expectations = user experiences.
Some user experiences have been better than others at setting these expectations, which ultimately match the consumer experience. In the GenAI domain, we’ve noticed that the winners’ growth is driven by what we call “Demonstration of Model Capabilities” (DMCs). Generative video and image models apps such as Midjourney, Sora, primarily benefited from DMC driven growth, through both their “explore” interfaces, onboarding, as well as some UI components.
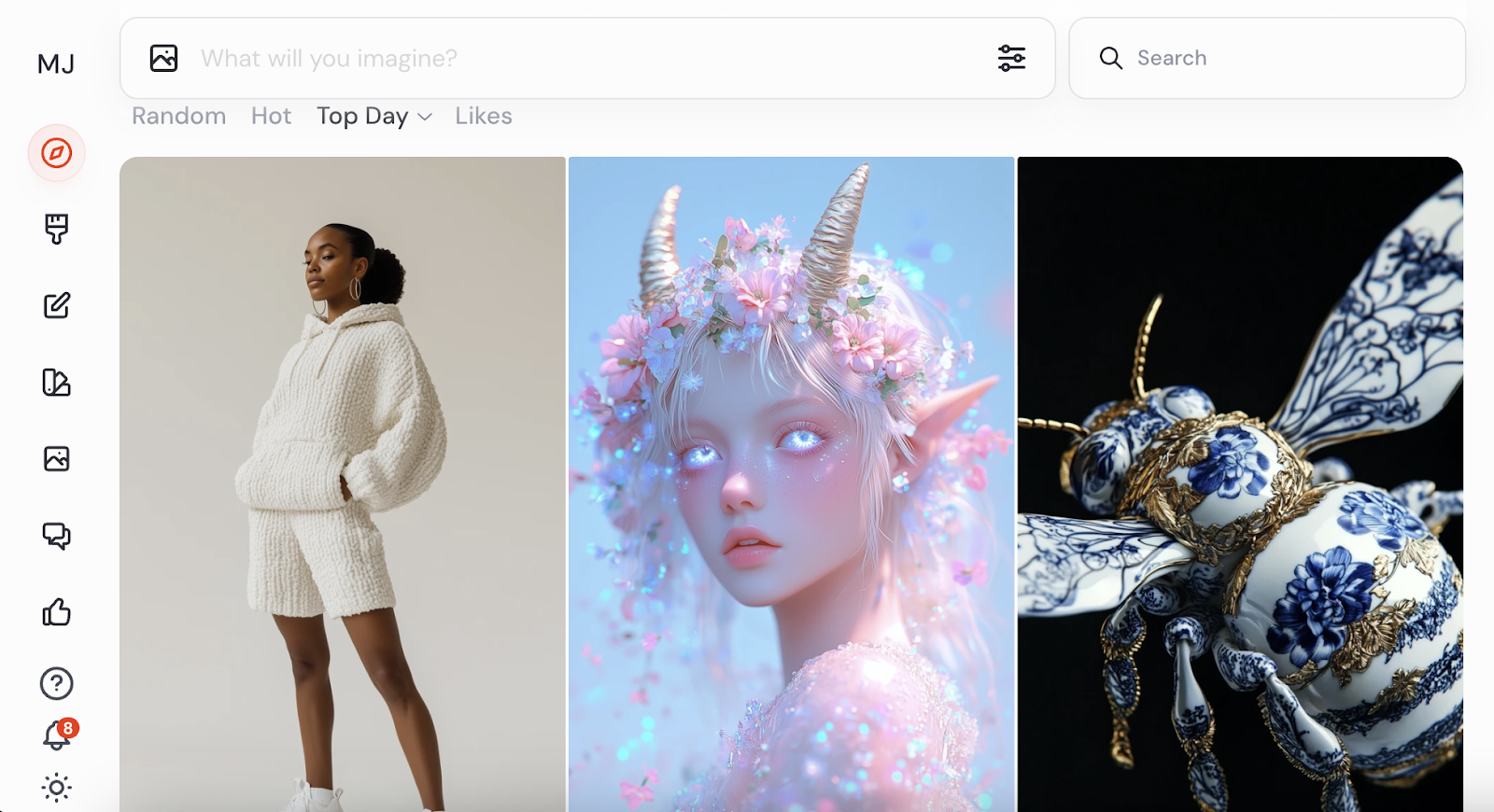
Midjourney (Gen AI Image Generator, Web Version): Strong Demonstrations of Model Capabilities (DMCs). When a user can quickly see the works being created by others that use this tool, it platforms clear user expectations that can realistically be met.
DMCs share to other users what could be made, up front - and make clearer customer promises in a cloudy market system dynamic marked by prototypes, experiments. One might contrast this to text based ChatGPT, Claude, et al, where a more minimalist interface is used, and these DMCs were cornered into GPTs, and the social web (e.g. DMCs across X - Twitter).
Current consumer AI experiences, across customer service agents, photo and video apps, and consumer LLMs, currently provide very limited DMCs to users. The result is that customer service agents may over promise, and under deliver. Again, reliability is created when a product consistently delivers what is consistently promised. Strong DMCs ground user expectations in what is truly possible. They also require less up front investigative effort from users.
Reliability, even within AI system integrators, is not up to par. A majority of revenues in the Generative AI consulting is currently derived from developing proof of concepts, and custom enterprise applications don’t appear to have been reliable enough yet. On the consumer side, the reliability of customer service chatbots in producing the intended outputs, strongly varies.
We believe, again, this can be resolved by simple design solutions: system indicators.
To address these product gaps, we have conceptualized a number of novel system indicators to remove frustrations from the user experience. They are based on the proposed guidelines in the development of generative AI guidelines proposed by Microsoft in 2019. The first two guidelines, “Make clear what the system can do”, and “Make clear how well the system can do what it can do”. DMCs effectively address both of these design guidelines.

Microsoft, 2019 [16]
Technology Windows, and the future
You may be asking: when are these changes coming? Venture fund nFX proposes “technology windows” - brief, optimal periods whereby startups can efficiently capitalize on emerging technologies, before costly competition arrives. Research firm Gartner, lists multimodal UX / multimodal interfaces as being 5 years away from the "plateau of productivity” [17, Gartner]. Our current brief assessment of the patent landscape also reveals trends in patent applications for ‘multimodal interfaces’ globally, suggesting that commercialization should follow between 3 - 7 years, supporting Gartner’s views [18, NDR]. The technology window seems to be wide open.
Where may these changes come from?
Our reading of a variety of scientific papers in the domains of Human Computer Interaction (HCI), involvement with the UX design community, as well as some correspondence with leaders such as Don Norman frequently brings us to the ecological sciences. Ecological terms such as “ecological validity”, “computing ecologies“, and “ecosystems” continue to fill the field. We believe that the industry's innovations in HCI and UX will come from both the theoretical approaches, and technical tools, currently deployed in ecology. This broadening of the discipline allows it to gain more influence. In Weiser’s important Scientific American article that popularized ubiquitous computing, he posits that natural environments contain much larger amounts of information than artificial ones, yet promises that value in these environments is more easily found.
Adaptive interfaces also doesn’t appear to be far away. Adaptive interfaces (highly personalized interfaces for particular tasks, with particular contexts, for users with unique preferences, and abilities). Google Deepmind Research Scientist Merredith Morris, writes in a recent 2025 ACM Interactions article, of a generated interface that is unique to every situation. The primary challenge is engineering the best model that is able to weigh both implicit and explicitly interactions, within an infinitely long tail of potential user contexts, preferences, which makes learning general principles difficult.
In Closing
Crafting an open and broad exploratory brief presents challenges in drawing definitive conclusions. However, this approach allows us to synthesize novel insights. Based on our exploration, we close with the following:
Americans are generally pessimistic about AI. Science Fiction is great for explaining, and examining Western user attitudes, conducting strategic foresight exercises, and producing inventive UX, UI, and Interaction Designs. Users want transparent AI interfaces, and transparent interfaces should display their capabilities up front. Trust related UX improvements can save in the low $X00’s of millions globally. Major UX discoveries for AI interfaces will come from the Ecological Sciences. Major UX inventions for AI interfaces will be experienced within 3 to 7 years [19, NDR].
We are a growing consumer behaviour research practice based in Toronto, established in 2019, primarily serving the tech industry. We are focused on discovering strong, resilient consumer insights through a holistic approach to research that fuses rigorous traditional research methods, novel patent pending research systems, as well as PhD level insights from neighbouring disciplines that bring fresh light into critical business concerns. If you’d like to explore how this future will impact you, or partner with us on UX, market, and consumer research and development, reach out to us at mark@nguyendigital.co. Thanks for your evaluation.
Citations
[1] Pew Research Center. Faverio, M., & Tyson, A. (2023, November 21). What the data says about Americans’ views of artificial intelligence.https://pewrsr.ch/3SOjvNZ
[2] Statista Report. Carollo, L. (2025, January 6). Top movie genres in the U.S. & Canada 1995–2024, by total box office revenue.
[3] NDR Research Report, 2024.
[4] Scientific American. Weiser, M. (1991). The computer for the 21st century. 265(3), 94–104.
[5] Information & Management, Legris, P., Ingham, J., & Collerette, P. (2003). Why do people use information technology? A critical review of the technology acceptance model. 40(3), 191–204.
[6] Personal and Ubiquitous Computing, Borsci, S., Malizia, A., Schmettow, M., van der Velde, F., Tariverdiyeva, G., Balaji, D., & Chamberlain, A. (2022). The Chatbot Usability Scale: The design and pilot of a usability scale for interaction with AI-based conversational agents. 26, 95–119. https://doi.org/10.1007/s00779-021-01582-9scirp.org
[7] Jakob Nielsen, LinkedIn Post, 2025.
[8] Borsci, S., Malizia, A., Schmettow, M., van der Velde, F., Tariverdiyeva, G., Balaji, D., & Chamberlain, A. (2022). The Chatbot Usability Scale: The design and pilot of a usability scale for interaction with AI-based conversational agents. Personal and Ubiquitous Computing, 26, 95–119. https://doi.org/10.1007/s00779-021-01582-9
[9] McKinsey & Company. Chui, M., & Yee, L. (2023). The economic potential of generative AI: The next productivity frontier. https://www.mckinsey.com/capabilities/mckinsey-digital/our-insights/the-economic-potential-of-generative-ai-the-next-productivity-frontier
[10] Nielsen Norman Group - 7 Heuristics of UX Design
[11] NeurIPS, 2023. Turpin, M., Michael, J., Perez, E., & Bowman, S. R. (2023). Language models don't always say what they think: Unfaithful explanations in chain-of-thought prompting. arXiv. https://doi.org/10.48550/arXiv.2305.04388
[12] Proceedings of the 54th Hawaii International Conference on System Sciences. Gerlings, J., Shollo, A., & Constantiou, I. (2021). Reviewing the need for explainable artificial intelligence (xAI). https://hdl.handle.net/10125/70768
[13] Patel, D., & Patel, S. (2024). How explainable artificial intelligence can increase or decrease clinicians' trust in AI applications in health care: Systematic review. JMIR AI, 1(1), e53207. https://doi.org/10.2196/53207
[14] Open AI. Shavit, Y., Agarwal, S., Brundage, M., Adler, S., O'Keefe, C., Campbell, R., Lee, T., Mishkin, P., Eloundou, T., Hickey, A., Slama, K., Ahmad, L., McMillan, P., Beutel, A., Passos, A., & Robinson, D. G. (2024). Practices for governing agentic AI systems. https://cdn.openai.com/papers/practices-for-governing-agentic-ai-systems.pdf
[15] IBM Research - In AI We Trust? Factors That Influence Trustworthiness of AI-infused Decision-Making Processes
[16] Microsoft, 2019.
[17] Gartner Report on Gen AI Hype Cycle, 2024.
[18] NDR Analysis: based on PatentScope (WIPO), Clarivate Derwent Innovation analysis, Various journals in HCI discipline (e.g. Computers in Human Interaction, ACM Journals related to HCI), and sample writing from the UX/Design/HCI Community on Medium.
[19] NDR Analysis: estimates based on McKinsey & Co 2023 Gen AI Market sizing * Expected adoption due to improved ease of use.